《Tensor Multi-Elastic Kernel Self-Paced Learning for Time Series Clustering》学术报告
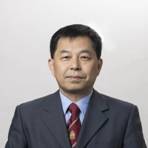
应郑州大学电气工程学院邀请,中国科学院自动化研究所张文生研究员莅临我校做学术报告,欢迎广大师生参加并交流讨论!
报告题目:Tensor Multi-Elastic Kernel Self-Paced Learning for Time Series Clustering
报告人:张文生 研究员
报告时间:2019年11月18日(星期一)下午14:00-15:30
报告地点:电气工程学院二楼大会议室
报告人简介:
Wensheng Zhang is currently a Professor and Vice Chief Engineer at Institute of Automation Chinese Academy of Sciences (CASIA). He is also a Chief Professor in Artificial Intelligence at the University of Chinese Academy of Sciences. His research focuses on artificial intelligence, statistical machine learning, intelligence analysis for big data and video/image understanding. He is a member of the General Expert Group of National Key R&D Program of China on "Cloud Computing and Big Data", and also a member of the General Expert Group of National Key R&D Program of China on "Internet of Things and Smart City". He is now an Expert of the National Natural Science Foundation of China (NSFC), and the Review Expert of National Science and Technology Awards. He is also the Vice Director of the Internet of Things Work Committee in China Instrument and Control Society (CIS), Vice Chairman of the Special Committee on Intelligent Service in the Chinese Association for Artificial Intelligence (CAAI) and Vice Chairman of the Special Committee on Intelligent Control in Chinese Association of Automation (CAA).
报告简介:
Time series clustering has attracted growing attention due to the abundant data accessible and extensive value in various applications. The unique characteristics of time series, including high-dimension, warping and the integration of multiple elastic measures, pose challenges for the present clustering algorithms, most of which take into account only part of these difficulties. We make an effort to simultaneously address all aforementioned issues in time series clustering under a unified multiple kernels clustering (MKC) framework. Specifically, we first implicitly map the raw time series space into multiple kernel spaces via elastic distance measure functions. In such high-dimensional spaces, we resort to the tensor constraint based self-representation subspace clustering approach, involving in the self-paced learning paradigm, to explore the essential low-dimensional structure of the data, as well as the high-order complementary information from different elastic kernels. The proposed approach can be extended to more challenging multivariate time series clustering scenario in a direct but elegant way. Extensive experiments on 85 univariate and 10 multivariate time series datasets demonstrate the significant superiority of the proposed approach beyond the baseline and several state-of-the-art MKC methods.